

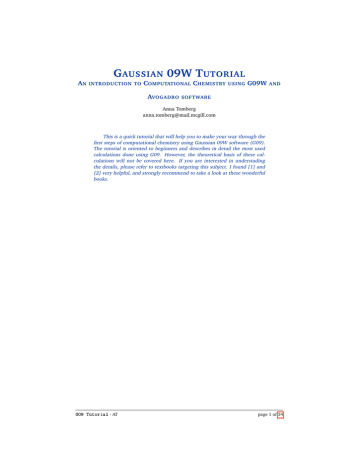

Now that we have worked through the necessary equations, we will think about how we can understand the two operations visually. Note that the new mean only depends on the conditioned variable, while the covariance matrix is independent from this variable. The diagonal of Σ \Sigma Σ consists of the variance σ i 2 \sigma_i^2 σ i 2 of the i i i-th random variable.Īnd the off-diagonal elements σ i j \sigma_\:) \\ The covariance matrix is always symmetric and positive semi-definite. Σ \Sigma Σ models the variance along each dimension and determines how the different random variables are correlated. The mean vector μ \mu μ describes the expected value of the distribution.Įach of its components describes the mean of the corresponding dimension. You can see an interactive example of such distributions in the figure below. The multivariate Gaussian distribution is defined by a mean vector μ \mu μ and a covariance matrix Σ \Sigma Σ. In particular, we are interested in the multivariate case of this distribution, where each random variable is distributed normally and their joint distribution is also Gaussian. They help to explain the impact of individual components, and show the flexibility of Gaussian processes.Īfter following this article we hope that you will have a visual intuition on how Gaussian processes work and how you can configure them for different types of data.īefore we can explore Gaussian processes, we need to understand the mathematical concepts they are based on.Īs the name suggests, the Gaussian distribution (which is often also referred to as normal distribution) is the basic building block of Gaussian processes. We will first explore the mathematical foundation that Gaussian processes are built on - we invite you to follow along using the interactive figures and hands-on examples. The mean of this probability distribution then represents the most probable characterization of the data.įurthermore, using a probabilistic approach allows us to incorporate the confidence of the prediction into the regression result. Gaussian processes offer an elegant solution to this problem by assigning a probability to each of these functions. įor a given set of training points, there are potentially infinitely many functions that fit the data.
GAUSSIAN SOFTWARE TUTORIAL SERIES
This is called regression and is used, for example, in robotics or time series forecasting.īut Gaussian processes are not limited to regression - they can also be extended to classification and clustering tasks. Their most obvious area of application is fitting a function to the data. They allow us to make predictions about our data by incorporating prior knowledge. Gaussian processes are a powerful tool in the machine learning toolbox. With this blog post we want to give an introduction to Gaussian processes and make the mathematical intuition behind them more approachable. Even if you have spent some time reading about machine learning, chances are that you have never heard of Gaussian processes.Īnd if you have, rehearsing the basics is always a good way to refresh your memory.
